Previously, in this series we discussed why Findability, Discovery, and Inspiration are vital for analyzing and understanding search quality in eCommerce. These search quality dimensions relate mainly to the items or products themselves. We now turn our attention to another dimension, defined as Search Quality in eCommerce Sellability.
What are the Three Pillars of Search Quality – a Recap
Let me articulate this as clearly as possible: Relevance and Discovery or Inspiration, in isolation, are insufficient to judge search quality for eCommerce. And this is why.
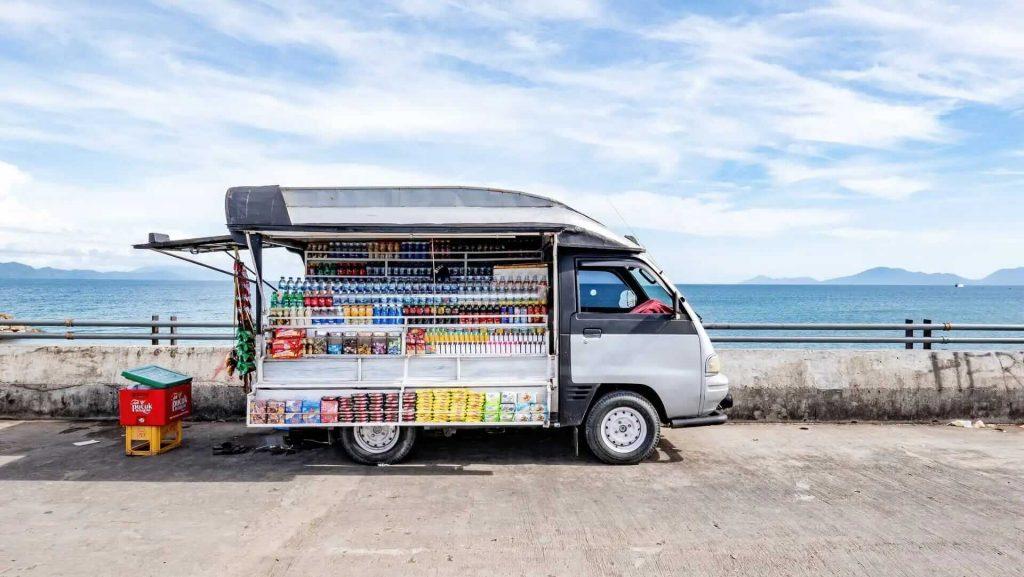
Even if still in the consideration phase, shoppers are often not simply looking for a product. And as a seller, you are not just offering products. The offer you make as a seller to a potential buyer is (almost) always a combination of a product and its more or less time-specific availability and price. Unfortunately, many people tend to forget or ignore this — most likely due to the added complexity. Still, it is indeed one of the most critical parts of the puzzle.
If you fail to consider this, I guarantee you will forfeit the full potential your business could achieve. This is true irrespective of whether you are lucky or hardworking enough to have built or bought the best search & discovery platform out there. Provided you are not selling a unique product or type of product(s), alternative offers will exist. Based on the incredibly high adoption rate of Google, Bing, Amazon, Alibaba, et al., shoppers are aware of alternative offers.
Side note: There is, even more, to consider (quality of service, branding, trust, you name it) which, potentially, all influence the buying decision and, more specifically, the price sensitivity of a prospective buyer. But these factors are either very hard to differentiate or quantify (measure) at scale, while product pricing and availability are not. That’s why, I’ll focus on the latter in this post.
eCommerce Search Quality – Product Sellability
Sellability is a compound of the words Sell and Probability. It describes the likelihood or probability that a specific product sells at a particular time if exposed to the shopper. For simplicity’s sake, let’s assume all product properties are static. A fair condition unless the product gets an upgrade or update. In this case, there are only three dimensions you the seller can influence: demand, availability, and price.
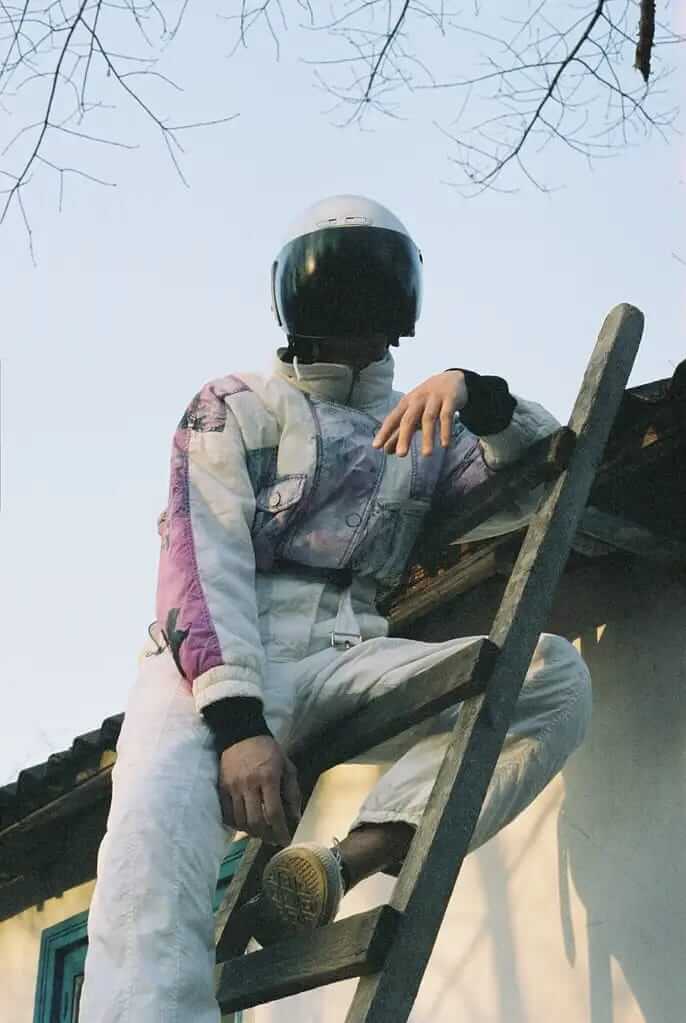
Demand as a Dimension of Sellability
Availability as a Dimension of Sellability
If a product is not in stock or unavailable, it’s pretty damn hard to sell. Therefore, regarding availability, there are also a couple of different scenarios to consider.
- You’re in the fortunate position to be the only one or one of the few who can sell a specific product. Maybe you have the exclusive right, or you are just faster in onboarding new products.
- The product is not yet in or out-of-stock.
- The product is generally available and in-stock.
Price as a Dimension of Sellability
Unfortunately, things get a bit more complicated when it comes to price. Demand-forecasting and price-optimization are two significant research areas of their own. However, using the following three scenarios, we can model the real world with reasonable accuracy. Please be aware: I assume the product is available. And as noted earlier, there are no distinct factors of competitive differentiation.
- Your offer has the lowest price compared with all alternative offers.
- Your offer has the highest price compared with all alternative offers.
- The price in your offer is quite close to your competition.
Real-World Sellability Calculation
Until now, we have reviewed the problem in theory only. Let’s switch gears and examine some actual data to check if we can spot any exciting patterns, correlations, or tendencies. These will help us better understand the problem we need to solve. We also hope to discover how sellability influences the results we measure and our Search Quality interpretation. Before we jump in, let me share how I gathered the data and why.
Sellability Calculation Methodology
First off, I spent some time researching products that at least three of our customers sell. After all it’s pretty useless attempt to understand sellability with data from just one shop. I looked at historical sales, prices, and availabilities over the last year.
Unfortunately, I’m not permitted to share any information about the sellers, their products, or prices. However, I can show is non-brand-specific information.
As a next step, I removed products for which we hadn’t enough data-points coverage over the last 12 months. And from the rest, I picked a small random sample-set for further analysis.
Additionally, I put the resulting products into four different price buckets (under €10, between €10-50, and above €100). I then filtered out all products, within the designated period, with a significant price variation, that resulted in a bucket change. I manually sorted these into an altogether separate bucket ensuring they would not be part of the current evaluation.
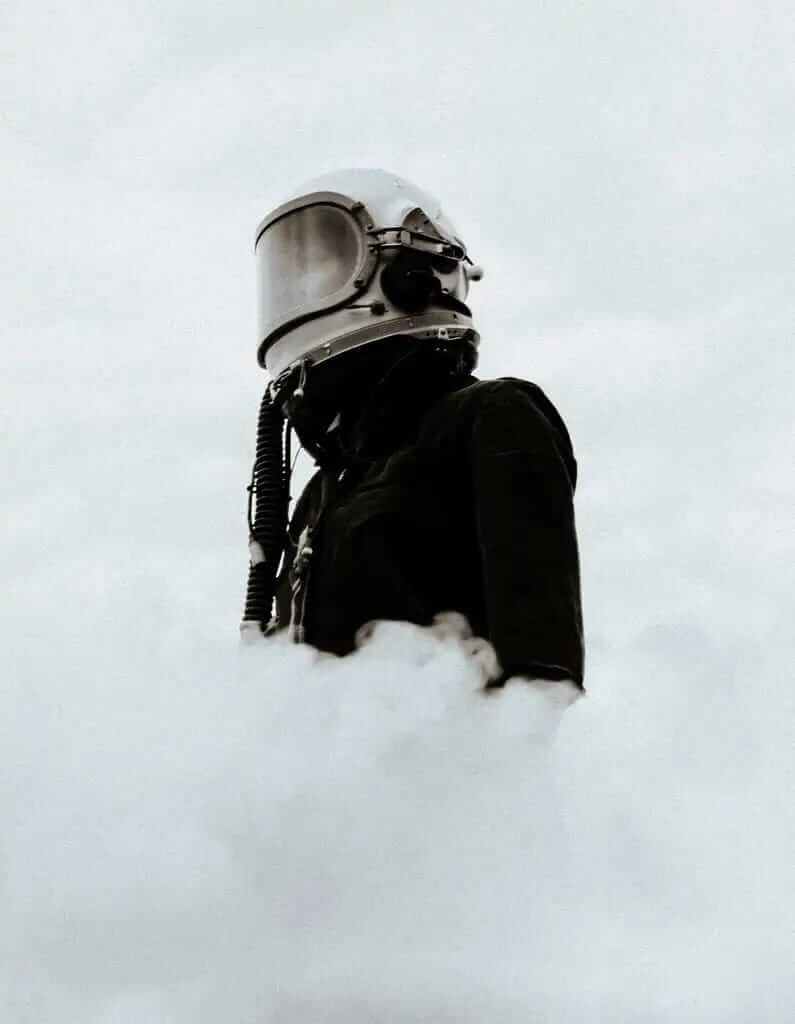
Sellability – How To Extract Useful Information from the Data
This gave me eleven unique products in the first, ten in the second, and fifteen in the third bucket. All products fulfilled the above criteria. Once I had the data, I mainly observed the influence of pricing and availability on the view2click, view2buy-ratio, and nDCG@20.
How To Leverage view2click and the view2buy-ratios
For the pricing, I decided to do the following. I wanted to evaluate how a shop and its competitor’s pricing influences the respective shop metrics. So, I calculated the percent difference in price between the shop in question and the minimum price of its competition.
The view2click-ratio is a straightforward yet compelling metric. It essentially gives you an idea of how attractive a product is for your audience. The closer this ratio gets to 1, the more attractive the product seems to be for your audience.
The view2buys-ratio is quite similar. It’s more explicit in terms of business value since it essentially measures how well a product sells. Once again, the closer this ratio gets to 1, the more sellable the product seems to be for your audience.
nDCG@20 and Your Search Quality Bias
Regarding nDCG@20 — Many companies use implicit feedback (Clicks and Carts) as signals to develop query-relevance judgments in eCommerce. Based on these judgments, they then run automated nDCG-evaluations. Much effort can and should be spent on methods to understand these signals’ correct conclusions. I will keep it straightforward though, since the effects I’m looking for will affect each method or model below.
- For a given query, we count the clicks and carts for every product in the result-set.
- For clicks, we assign a weight of 1, and carts a weight of 3. Then calculate the weighted sum for each query/product pair and assign it to the variable interactions.
- Now we do a maximum-normalization. We take the maximum number of interactions for every query and divide all the other product interactions by this value. You can skip this normalization, or you could and should use other normalization functions. Let’s stick with this one for simplicity’s sake. In this way, all interaction values for our query/product tuples are normalized within a range between (0,1).
- The next thing we have to do is map the interaction values into the judgment space. There might exist infinite methods to do this, but I will again keep it straightforward. Let’s say we are going to assign judgments from (1,5). This results in 5 different judgment values that we could assign to a query/product pair. So let’s divide the interaction value range into five equal-sized buckets. For example, query/product pairs with an interaction value below 0.2 would map to judgment value 1, and so on.
- Once we have this mapping in place, we can calculate an optimal product ranking based on the judgments.
- Now we compare our optimal product ranking based on judgments with the observed click positions on the first 20 results and thus arrive at the nDCG@20.
nDCG@20 a Practical View
Suppose you work for a company that gathers implicit feedback (Clicks and Carts). It uses this feedback as signals to develop query-relevance judgments for eCommerce. They then perform automated NDCG-evaluations based on these judgments. If this sound like you, have a closer look at the next part.
With everything defined, I went on and calculated the different values for which I was looking. I did this for all three shop-competitor combinations and averaged the results, printing them in the following chart.
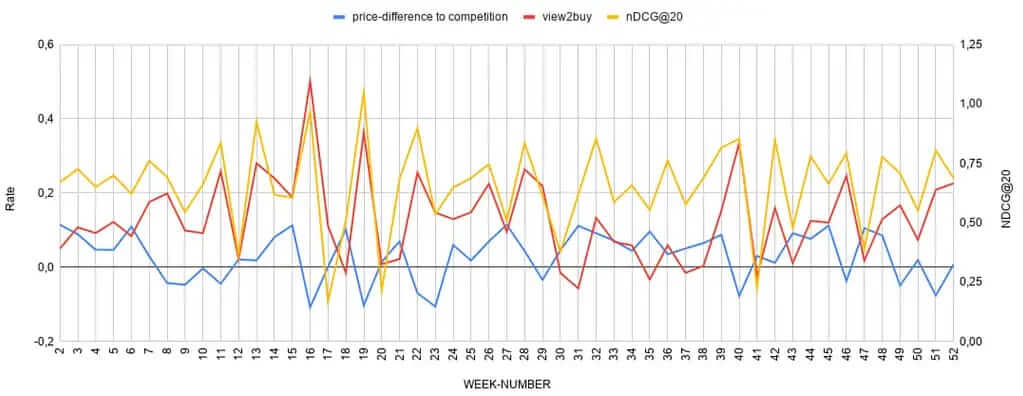
The issue here is that changes in price or availability can significantly influence the user’s contextual relevance. This is true even if the textual and or semantic relevance between query and product hasn’t changed at all. This directly affects the click and cart probabilities.
You may have spotted that I only include the data for the first price bucket. If you are interested in how the charts look like for the other buckets, PM me 🙂
Conclusion
This is the final entry for the Three Pillars of Search Quality in eCommerce Search series. I hope that the content I created helps you during your journey. To discover the perfect balance between what the seller wants to sell and what the users want to buy.
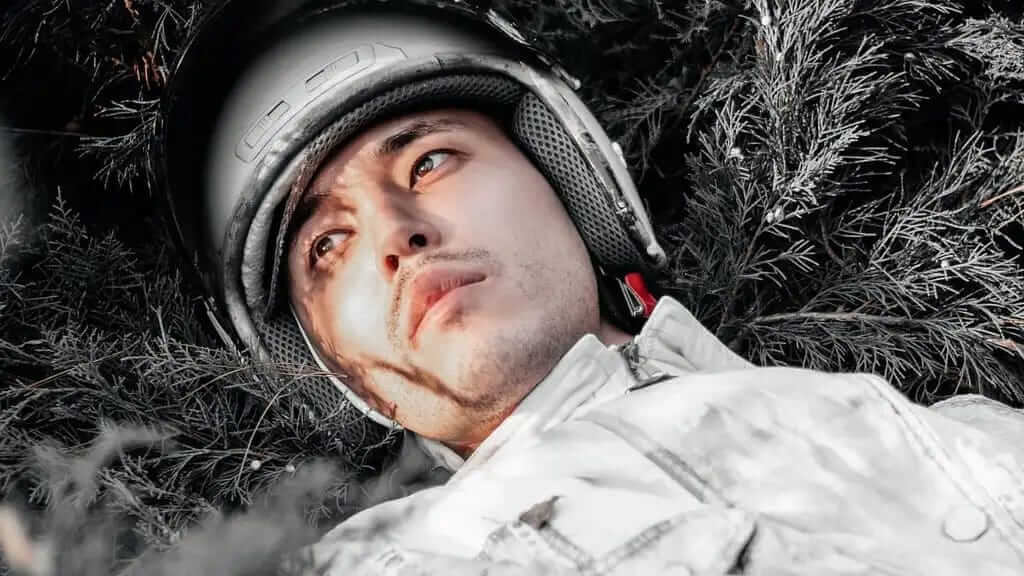
Furthermore, if you’ve made it this far, you’re without excuse if you’re ever found stuck in strategies that never venture beyond findability improvements. You’re now equipped with the knowledge necessary to begin balancing the optimization of your discovery and inspiration journeys, against the underlying dimension of sellability.
Final words: It’s no trivial task to fix these types of bias. I understand that. However, over-simplifying the problem and ignoring the facts won’t help you differentiate from the competition. There is no way around it. Offering an outstanding shopping discovery experience means taking external factors (like market trends, or competitor pricing) into account.
Good Luck!