One of the biggest causes of website failure is when users simply can’t find stuff on your website. The first law of e-commerce states, “if the user can’t find the product, the user can’t buy the product.”
Why is Measuring and Continuously Improving Site-Search so Tricky?
This sentence seems obvious and sounds straightforward. However, what do “find” and “the product” mean in this context? How can we measure and continuously improve search? It turns out that this task isn’t easy at all.
Current State of Search Relevancy in E-Commerce:
When I talk to customers I, generally, see the following two main methods to measure and define KPIs against the success of search: relevancy, and interaction and conversion.
However, both have flaws in terms of bias and interpretability.
We will begin this series: Three Pillars of Search Relevancy, by developing a better understanding of “Findability”. But first, let’s begin with “Relevancy”.
Search Relevancy:
Determining search result relevance is a massive topic in and of itself. As a result, I’ll only cover this topic with a short, practical summary. In the real world, even in relatively sophisticated teams, I’ve only ever seen mainly three unique approaches to increase search relevancy.
- Explicit Feedback: Human experts label search results in an ordinal rating. This rating is the basis for some sort of Relevance Metric.
- Implicit Feedback: Various user activity signals (clicks, carts, …) are the basis for some sort of Relevance Metric.
- Blended Feedback: The first two types of feedback combine to form the basis for a new sort of Relevance Metric.
In theory, these approaches look very promising. And in most cases, they are superior to just looking at Search CR, Search CTR, Search bounce, and Exit rates. However, these methods are heavily biased with suboptimal outcomes.
Explicit Feedback for Search Relevancy Refinement
Let’s begin with Explicit Feedback. There are two main issues with explicit feedback. First: asking people to label search results to determine relevance, oversimplifies the problem at hand. Relevance is, in fact, multidimensional. As a result, it needs to take many factors into account, like user context, user intent, and timing. Moreover, relevance is definitely not a constant. For example, the query “evening dress”, may offer good, valid results for one customer, and yet, the very same list of results can be perceived as irrelevant for another.
Since there is no absolute perception for relevancy, it can’t be used as a reliable or accurate search quality measurement.
Not to mention, it is almost impossible to scale Explicit Feedback. This means only a small proportion of search terms can be measured.
Implicit Feedback for Search Relevancy Refinement
Moving on to Implicit Feedback. Unfortunately, it doesn’t get a lot better. Even if a broad set of user activity signals are used, as a proxy for Search Quality, we still have to deal with many issues. This is because clicks, carts, and buys don’t take the level of user commitment into account.
For example, someone who had an extremely frustrating experience may have made a purchase out of necessity and that conversion would be counted as successful. On the other hand, someone else may have had a wonderful experience and found what he was looking for but didn’t convert because it wasn’t the right time to buy. Perhaps they were on the move, on the bus let’s say. This user’s journey would be counted as an unsuccessful search visit.
But there is more. Since you only receive feedback on what was shown to the user, you will end up at a dead-end. This is not the case, however, if you have some sort of randomization in the search results. This means that all other results for a query, that have yet to be seen, will have a zero probability of contributing to a better result.
Blended Feedback for Search Relevancy Refinement
In the blended scenario, we combine both approaches and try to even out their short-comings. This will definitely lead to more accurate results. It will also help to measure and improve a larger proportion of search terms. Nevertheless, it comes with a lot of complexity and induced bias. This is the logical outcome, as you can only improve results that have been seen by your relevancy judges or customers.
Future State — Introducing Findability as a Metric
I strongly believe that we need to take a different approach to this problem. Then “relevance” alone is not a reliable estimator for User Engagement and even less for GMV contribution.
In my humble opinion, the main problem is that relevance is not a single dimension. What’s more, relevance should instead be embedded in a multidimensional feature space. I came up with the following condensed feature space model, to make interpreting this idea somewhat more intuitive.

Once you have explored the image above, please let it sink in for a while.
Intuitively, findability is a measure of the ease with which information can be found. However, the more accurately you can specify what you are searching for, the easier it might be.
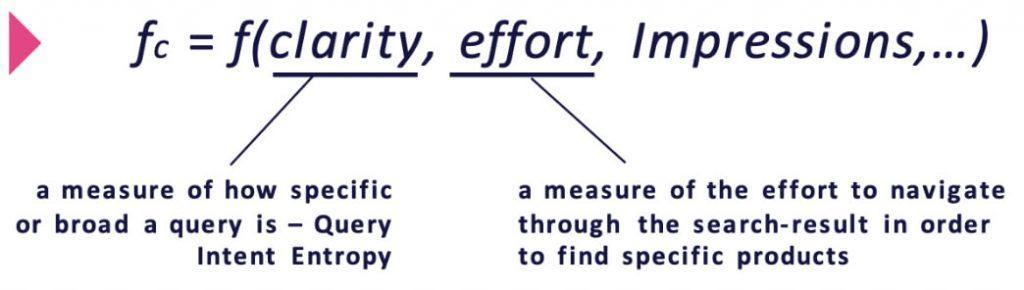
Findability – a Break-Down
I tried to design the Findability feature (measure) to do exactly one thing extremely well. Pointedly, to measure the clarity, effort, and success in the search process. Other important design criteria for the Findability score were that it should:
a) not only provide representative measures for the search quality of the whole website, but also
b) for specific query groups and even single queries to be able to optimize and analyze them.
Findability not only tries to answer, but goes a step further to quantify the question.
Findability as it Relates to Interaction, Clarity, and Effort
- “Did the user find what he was looking for?” — INTERACTION
it also tries to answer and quantify the questions
- “Was there a specific context involved when starting the search process?”. “Was the initial search response a perfect starting point for further result exploration?” — CLARITY
and
- “How much effort was involved in the search process?” — EFFORT
Appropriately, instead of merely considering whether a result was found and if a product was bought, we also consider whether the searcher had a specific or generic interest. Additionally, things like whether he could easily find what he was looking for, and if the presented ranking of products was optimal, provide valuable information for our findability score.
Intuitively, we would expect that for a specific query, the Findability will be higher if the search process is shorter. In other words, there is less friction to buy. The same applies to generic or informational queries, but with less impact upon Findability.
We do this to ensure seasonal, promotional, and other biasing effects are decoupled from the underlying search system and its respective configuration. Only by trying to decouple these effects, is it possible to continuously optimize your search system in a systematic, continuous, and efficient way respective to our goals to:
- increase the customer experience (to increase conversions and CLTV)
- increase the probability of interaction with the presented items
- increase the success rate of purchase through search
Building the Relevance and Findability Puzzle
To quantify the three different dimensions clarity, effort, and interaction we are going to combine the following signals or features.
Clarity – as it Relates to Findability:
In this context, clarity is used as a proxy for query intent type. In other words, information entropy. For example, in numerous instances, customers issue quite specific queries. For example: “Calvin Klein black women’s evening dress in size 40”. This query describes what they are looking for. For this type of query, the result is pretty clear. However, there is a significant number of examples where customers are either unable or unwilling to formulate such a specific query. On the other hand, the query: “black women’s dress”, leaves many questions open. Which brand, size, price segment? As a result, this type of query is not clear at all. That’s why clarity tries to model the query and deliver specificity.
Features |
---|
Query Information Entropy |
Result Information Entropy |
Effort – as it Relates to Findability:
Effort, on the other hand, attempts to model the exertion, or friction, necessary for the customer to find the information or product for the complete search process. Essentially, every customer interaction throughout the search journey, adds a bit of effort to the overall search process, until he finds what he is looking for. We must try to reduce the effort needed as much as possible, as it relates to clarity, since every additional interaction could potentially lead to a bounce or exit.
Features (Top 5) |
---|
Dwell time of the query |
Time to first Refinement |
Time to first Click |
Path Length (Query Reformulation) |
Click Positions |
Based on these features, it is necessary, in our particular case, to formulate an optimization function that reflects our business goals. Our goal is to maximize the expected search engine result page interaction probability while minimizing the needed path length (effort).
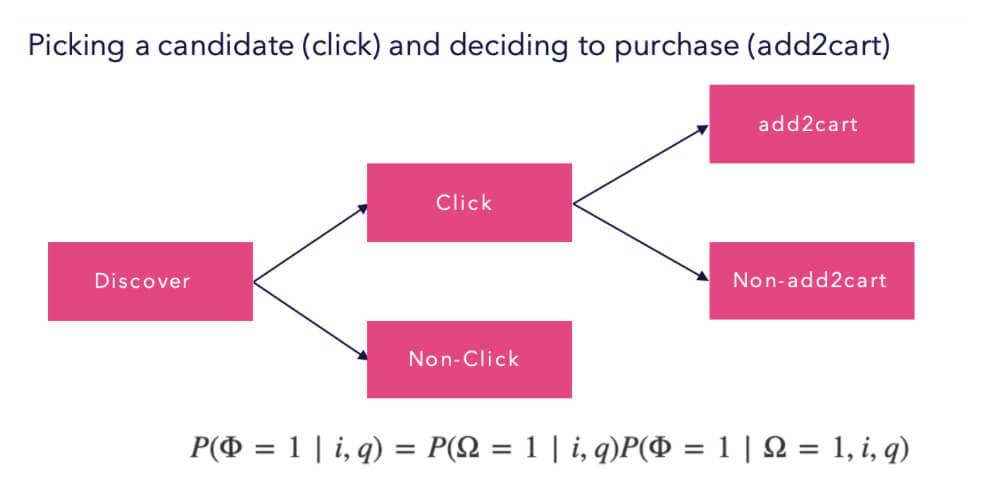
The result of our research is the Findability metric (a percentage value between 0-100%), where 0% represents the worst possible search quality and 100% the perfect one. The Findability metric is part of our upcoming search|hub Search Insights Product, which is currently in Beta-Testing.
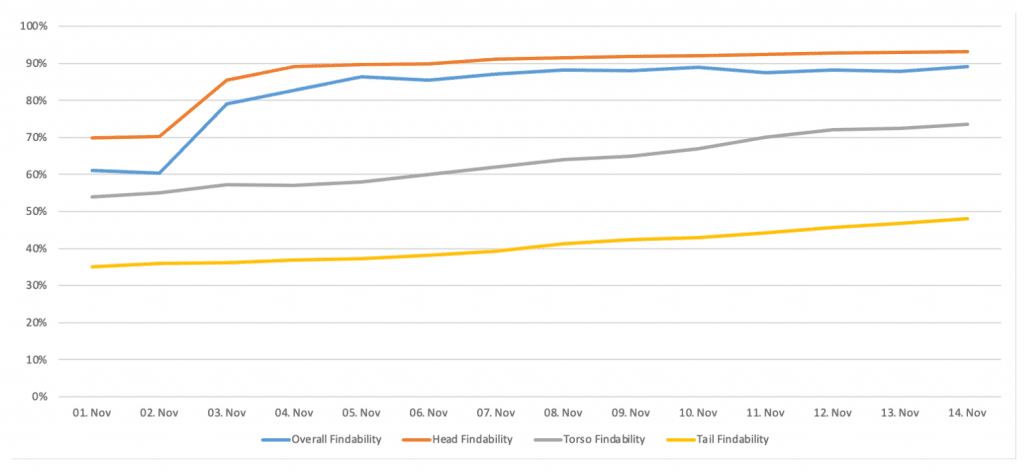
I’m pretty confident that providing our customers easier to understand and more resilient measures about their site search, will allow them to improve their search experiences in a more effective, efficient, and sustainable way. Therefore, the Findability should provide a solid and objective foundation for your daily and strategic optimization decisions. Simultaneously, it should give you an overview of whether your customers can, efficiently, interact with your product and service offerings.